AI to predict critical care for patients with COVID-19
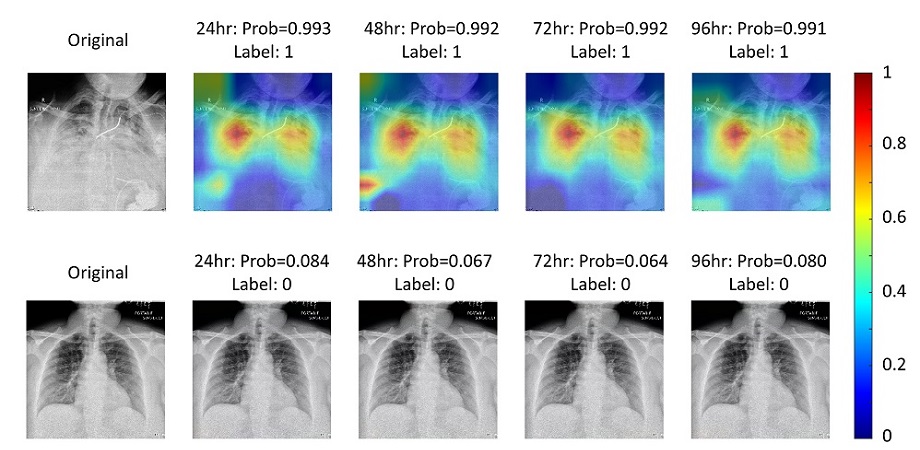
The COVID-19 pandemic dealt a huge blow to healthcare systems and highlighted their major shortcomings. As of June 2023, there have been over 760 million confirmed cases of COVID-19, with almost 7 million deaths worldwide. During the major COVID-19 outbreaks, hospitals often had their intensive care units (ICU) running at full capacity for providing invasive mechanical ventilation to patients who were diagnosed as positive for COVID-19. These ICUs often operated with insufficient staff and intubation equipment.
One way to mitigate such problems is to accurately predict the prognosis of patients who test positive for COVID-19. Doctors generally use chest X-ray radiography (CXR) images to assess the condition of patients. By analyzing signs of pneumonia in these images, they can infer if the patient is likely to need admission to the ICU soon. In turn, this can help with optimal allocation of hospital resources. Unfortunately, this process is labor intensive, time consuming, and suffers from variability in diagnoses, which is a major issue especially during large outbreaks.
But what if artificial intelligence (AI) gave us a helping hand? In a recent study published in the Journal of Medical Imaging, a team of researchers from the Department of Radiology of the University of Chicago has developed a deep learning-based model that can predict if a patient will need intensive care by analyzing their CXR images.
One of the defining features of the proposed approach was the use of a technique called “transfer learning.” Developing deep learning models for medical imaging applications is especially challenging because of the sheer amount of annotated data required for training. Thus, instead of training a model from scratch with millions of images, one can use transfer learning to pass knowledge from a pretrained model to another model. The idea is to fine-tune the receiving model using a dedicated dataset so that the “expertise” of the previous model can be leveraged for a new task. For example, with transfer learning, a model trained to detect a specific disease in magnetic resonance images can serve as the basis for another model aimed at detecting a different disease.
Utilizing this strategy, the researchers employed a sequential transfer learning process to develop their final model. They first fine-tuned a large model, pretrained on ImageNet with 1.2 million natural images, using CXR images from a National Institutes of Health dataset to detect 14 different diseases. After that, the researchers refined this model using a dataset from the Radiological Society of North America to detect pneumonia. Lastly, they fine-tuned it using an in-house dataset, which contained 6,685 CXR images from 3,998 patients with COVID-19.
The developed AI model could predict whether a patient with COVID-19 would need intensive care within 24, 48, 72, and 96 hours of the CXR exam with a good degree of accuracy. In an independent in-house test set (1,672 CXR images from 1,048 patients), it achieved an area under the receiver operating characteristic curve of 0.78 when predicting the need for intensive care 24 hours in advance, and at least 0.76 for 48 or more hours, with patients identified by the model as high risk being almost five times as likely to require intensive care. Interestingly, the proposed model’s performance was comparable to similar existing models, even though it relied only on CXR images instead of a combination of images and clinical data.
Overall, this model could fill an important gap in clinical practice. While many machine learning models were developed to diagnose COVID-19, few were designed to predict the prognosis of patients. The proposed model, however, could play an essential role in supporting clinical decision-making and resource management, which in turn would improve the quality of care received by patients. Notably, the researchers are already working on improving this model in various ways. These include training the model with CXR images gathered at multiple institutions, incorporating relevant clinical variables into the model, extending the model to include lung opacities caused by related diseases, and adding image segmentation and preprocessing steps.
Here’s hoping that these efforts surely lead to better clinical outcomes for patients with COVID-19 during a pandemic!
Read the Gold Open Access article by Li, Drukker, et al., “Predicting intensive care need for COVID-19 patients using deep learning on chest radiography,” J. Med. Imag. 10(4) 044504 (2023), doi 10.1117/1.JMI.10.4.044504
Enjoy this article? Get similar news in your inbox |
|