Deep learning: A powerful tool for biophotonics in labs and clinics
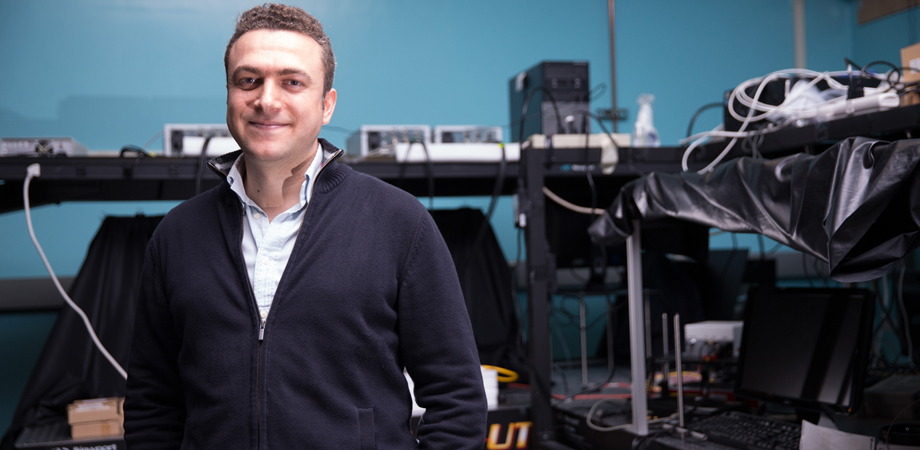
The increasing importance of deep learning (DL), artificial intelligence (AI) and related computational methods to biophotonics and clinical practice was highlighted during a BIOS plenary event at SPIE Photonics West in January.
Aydogan Ozcan of UCLA chaired the session and is also received the Dennis Gabor Award in Diffractive Optics in recognition of his accomplishments in diffractive wavefront technologies, a field of central importance to the breakthroughs under discussion.
“The potential for deep learning to assist in image analysis and direct diagnosis is becoming well known,” commented Ozcan. “My topic at this plenary session is more focused on how DL can reconstruct images with better resolution or enable image transformations that are beyond our current understanding of physical models in computational microscopy. This new way of thinking can help us transform the existing tools used in, for example, histology.”
Traditional histology has involved the sectioning of tissue samples into thin layers for staining with specific chemical markers, in order to reveal the cells of interest. The drawback is that this takes time to carry out, requires the individual attention of specialist technicians, and moves the activity away from the immediate clinical treatment of a patient.
“Instead of sending tissues into a histology lab where a human technician works with chemicals and labels, DL could let us take label-free autofluorescence images of those tissue sections without any external agents and apply trained neural networks to mimic the stained version of the same tissue image,” said Ozcan. “You could potentially replace a whole field of histology with appropriate neural network models.”
Virtual staining has the advantages of being fast, cheap and repeatable. Courtesy of Aydogan Ozcan, UCLA.
Bypassing the chemistry in this way will make the process inherently faster. Instead of waiting for a day or a week, the result will be seen on-demand in minutes, making the overall workflow more cost effective. It should also help to democratize access to histology, removing the requirement for samples to be treated at fewer well-resourced medical centers.
“We call it call it virtual staining, and it has the advantages of being fast, cheap and repeatable,” Ozcan noted. “Chemical staining is a delicate procedure, especially for immunohistochemical staining for certain cancers, and pathologists know better than anyone that if you send 100 biopsies from 100 patients to a lab for advanced staining, then 30 percent of the staining will not lead to a definitive result. Pathologists see the results and know immediately that the staining has failed, or the tissue is distorted, and a week or two may have been lost.”
Environmentally friendly
As well as speed and sensitivity, virtual staining should lead to the elimination of unnecessary biopsies. A significant number of patients have to be recalled for a repeat procedure, after the original biopsy fails to deliver a definitive verdict.
“Traditional staining methods are destructive; they deplete the tissues, and the stained materials cannot then be reused,” said Aydogan Ozcan. “With virtual staining, we don’t do anything to the tissue sample except capture an image of it. I can carry out further analysis or repeat an earlier one because we have not destroyed or lost the tissues, another major advantage.”
Since the tissues are still intact and unchanged, another different molecular analysis or virtual chemical staining can be carried out on the same sample, something beyond the capabilities of traditional methods. At present, if a diagnostician wishes to examine tissues with different contrasts or stains then fresh sections of tissue must be obtained, a methodology accepted in conventional microscopy but which deep learning can get around.
“The impact of deep neural nets as a means to perform some unique transformations within the microscopy optical microscopy domain will be significant, not least from the perspective of virtual staining and the concept of mimicking the staining process,” concluded Ozcan. “Plus, it’s a green technology. The staining processes today wastes millions of gallons of water a year globally, and the staining chemicals can be very toxic. Virtual staining is dye free and we do not have to create waste, making it attractive from the perspective of environmental protection and sustainability too.”
Computational imaging without a computer
In his plenary presentation Ozcan will also discussed what is essentially the opposite side of the same coin. If deep learning can enable new functions for microscopy by taking existing optical images into the digital domain and processing them there, can optics itself do any from of similar processing operations, without the need for external neural nets or computer graphics processing units (GPUs) in the first place?
This is the field of all-optical image reconstruction engines — computational imaging without a computer. Ozcan’s UCLA group has made great progress in diffractive computing, in which a sequence of fabricated diffractive surfaces act on light from objects hidden behind diffusing media and reconstruct images of those objects from the randomly scattered input. AI approaches can be involved in creating and training the diffractive surfaces, but the ultimate image reconstruction then takes place without numerical processing. The diffractive volume itself computes a stable image as light penetrates through it and gets diffracted.
Left: Diffractive image reconstruction, in which a sequence of fabricated diffractive surfaces act on light from objects hidden behind diffusing media. Right: Images of those objects are reconstructed from the randomly scattered input. Courtesy of Aydogan Ozcan, UCLA.
“These surfaces are materials that we engineer layer by layer, like an indivisible deck of cards at the wavelength scale,” said Ozcan. “Think of it as a very thin and transparent stamp with features engraved into it at the microscale, which can take information in the analogue wave domain and carry out some form of processing operation on that information as it diffracts through the material.”
Such an approach might be a new solution to the classic optics problem of seeing through opaque or scattering media, greatly of interest for defense, consumer and medical applications. Methods to do so have already improved drastically in recent years, but involve computers and significant processing power, as Ozcan explained.
“At present you will have an image capture operation, followed by digitization and perhaps upload to a cloud for storage, where a GPU with a specific neural network or another algorithm processes the image to see through the diffuser. It’s slow and it stores unnecessary information, and I want to change all that. The diffractive approach can give you the solution in picoseconds as the light is transmitted through the very thin optical element, which means you don’t need to even store it each time and you can see the reconstructed image on the fly.”
The same technique could have a major impact on techniques now based on quantitative phase imaging (QPI), presenting an all-optical solution to the traditionally complicated and computationally demanding problem of phase recovery.
A pyramid of opportunities
As in all fields where computation is involved, advances in digital operations place new emphasis on the need for accurate and clean data to be available as the input; otherwise it’s a case of rubbish in equals rubbish out.
“Neural networks are known to hallucinate,” said Aydogan Ozcan. “In pathology we obviously do not want to diagnose a cancer from a hallucinated image, only real cancers from real data. It’s logical that virtual staining of this kind could in fact deceive diagnosticians since it seems real and might perhaps look like malignancy or cancer.”
Setting up the neural networks that carry out digital staining will be the critical parameter to avoid these ambiguities, using the best authentic microscopy images of tissues and microstructural features to do the training.
Setting up neural networks to carry out digital staining is the critical parameter to avoid ambiguous outputs. Courtesy of Aydogan Ozcan, UCLA.
Once optimized, virtual staining will open up what Ozcan terms a pyramid of opportunities, probably starting in applications away from the highly regulated sector of primary patient care. Many millions of tissue samples are created and stained in universities for use in animal research and toxicology studies, or by pharmaceutical companies using animal models to understand the efficacy of drugs, sectors where the efficiencies of virtual staining can be readily exploited.
“At the top of the pyramid is primary diagnosis and treatment of cancer or other diseases, which is naturally FDA regulated,” Ozcan commented. “We believe the quickest impact for virtual staining technology is going to be elsewhere, in toxicology studies for pharma and the research market. Teleconsultation will also be a big factor. A lot of doctors in the West are already consulted for secondary opinions by colleagues in other parts of the world. This is not FDA regulated, believe it or not, because there is already a primary workflow that gave the initial decision. Virtual staining technology could be a valuable addition to these teleconsultation procedures.”
A practical advantage could also arise following the continuing effects of Covid-19 on supply chains, many of which remain weakened or broken around the world. Some of the factories producing chemicals for histology staining were shut down for months, pushing end users to think about other ways to continue their work, examine their samples, or treat their patients.
“PictorLabs, a spin-out from my group at UCLA, launched in December 2022 after raising $18.8 million in funding to commercialize an AI-powered virtual staining platform,” said Ozcan. “Covid-19 has made the older technologies that rely on established supply chains become antique and a pain point for businesses, so the commercialization path is accelerating.”
Tim Hayes is a freelance writer based in the UK. He was previously industry editor of optics.org and Optics & Laser Europe magazine. This article originally appeared in the 2023 SPIE Photonics West Show Daily.
Enjoy this article? Get similar news in your inbox |
|